Today, as a society, we face several global challenges such as climate change, food and water scarcity, and global health pandemics. A major bottleneck to enabling next-generation technologies is the lack of efficient discovery tools for advanced functional materials that are synthesizable and practically usable. This inefficiency often results from the overwhelmingly large space of candidate materials and their processing conditions, which are sparsely observed. Conventional trial-and-error-based search approaches are frequently biased by our chemical intuition and tend to identify new materials similar to those already known to perform well, potentially leading to the discovery of suboptimal materials. Additionally, the high cost associated with experimental characterization and first principles quantum mechanical calculations restricts the size of available datasets. In this talk, I will discuss computational tools that integrate data science, machine learning, and quantum chemistry calculations to enhance material modeling and discovery capabilities. Firstly, I will introduce PAL 2.0, a method that combines physics-informed surrogate models with Bayesian optimization (BO) to expedite materials discovery. The key contributing factor of this framework is the creation of a physics-based hypothesis using XGBoost and Neural Networks. Some applications of PAL 2.0 that I will discuss include perovskite solar cells, thermoelectric semiconductor materials, and covalent organic frameworks. I will briefly touch upon the application of PAL 2.0 in closed-loop materials discovery frameworks to accelerate the identification of high-hardness materials for space actuation applications and Mg alloys for biological purposes. Additionally, I will highlight my work on modeling hypersonic environments and materials discovery for extreme conditions, utilizing tools from fluid dynamics, deep operator learning, and molecular dynamics. Finally, I will briefly discuss my work on multi-scale material modeling and feature selection. Overall, my talk will underscore the application of cutting-edge data science and machine learning tools to accelerate material discovery, addressing some of the most challenging global issues in energy, sustainability, and healthcare.
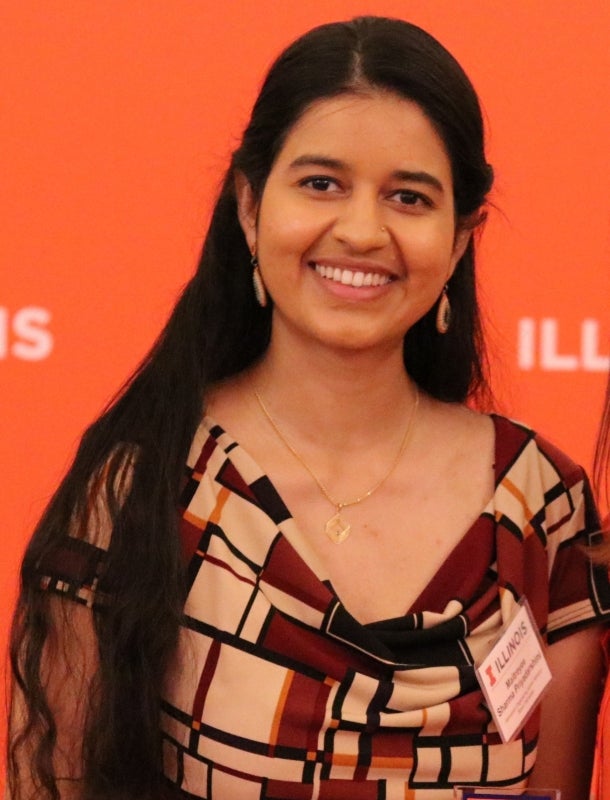
Dr. Maitreyee Sharma Priyadarshini is a Postdoctoral Research Associate with Prof. Paulette Clancy and Prof. Rigoberto Hernandez at Johns Hopkins University. She currently works on developing computational material discovery algorithms and multi-scale material models for energy and space applications. Before this, Maitreyee completed her Ph.D. in Aerospace Engineering at the University of Illinois, Urbana-Champaign, advised by Prof. Marco Panesi. Her thesis work focused on developing a computational framework to predict and mitigate heating on spacecraft surfaces accurately. Maitreyee’s work has been recognized by multiple awards and honors, including the Distinguished Young Scholars Best Speaker Award (University of Washington), Aerospace Engineering Alumni Advisory Board Fellowship (UIUC), Rising Stars in Aerospace (MIT), and Mavis Future Faculty Fellowship (UIUC). She is firmly committed to enhancing diversity, equity, and inclusion in STEM through mentoring, outreach, and service activities. Apart from research, she loves dancing and teaching dance. She has trained in Bharatnatyam, an Indian classical dance form, for over 20 years. She also enjoys practicing yoga and swimming.