Designing polymer membranes with high gas permeability and selectivity remains a grand challenge for energy, the environment, and economic sustainability. Increasing both the selectivity and permeability is a difficult constrained design problem for polymer membranes due a trade-off between these two properties. The complexity of chemical composition and morphology of polymers makes this design problem especially hard with trial-and-error or intuition-based strategies. In this talk, I will present a machine learning driven genetic algorithm to tackle the design problem of polymer membranes for CO2 separation from N2 and O2. Using literature data of permeability for three gasses - CO2, N2, and O2 - I constructed multiple ML models with different fingerprints to predict all three-gas permeability as well as the CO2/N2 and CO2/O2 selectivity values. Then, I employed a genetic algorithm to design new polymers and evaluated their performance with respect to the upper bounds using our machine learning models. I was able to identify new polymer membranes that are promising for both CO2/N2 and CO2/O2 separations. The framework identified here can be used to design polymer membranes for any application, where there is a constrained optimization problem. Finally, I will outline the strengths and limitations of this approach for any design problem, as well as the imminent challenges and opportunities with using machine learning guided data-driven inverse design of polymer membranes.
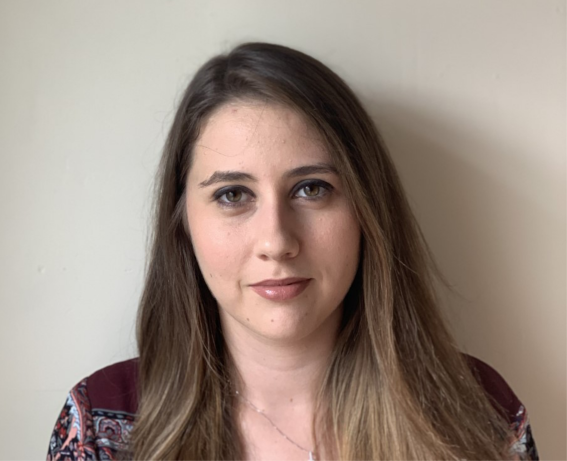
Yasemin Basdogan is currently a postdoctoral researcher working with Prof. Zhen-Gang Wang and a Resnick Research Fellow in Chemical Engineering at the California Institute of Technology. She received her PhD in Chemical Engineering at University of Pittsburgh, advised by Dr. John A. Keith, where she was awarded with the Dr. James M. Coull Memorial Award, given to the best PhD student, and the AIChE CoMSEF Graduate Student Award. She received her B.S. in Chemical Engineering from Koç University in Turkey, with four years of undergraduate research experience on gas storage in Metal Organic Frameworks. On the strength of her undergraduate research, she received a Fulbright Scholarship. Yasemin’s current research focuses on designing polymer membranes for CO2 separation through equation of state calculations, molecular dynamics simulations, and machine learning (ML) techniques. She has built a ML-driven genetic algorithm, for high throughput screening and ML-assisted polymer engineering, that got funded from the Resnick Sustainability Institute. This work resulted in multiple new polymers that perform better than the state-of-the-art industrial polymers. Her past research focused on modeling homogeneous reaction mechanisms and ion solvation environments using quantum chemistry and ML. She has built a framework to study solvated reaction mechanisms that has been successfully implemented for multiple reactions.